CST(Class-Shape Transformation)法を使用して翼型の形状を生成したり、既存の翼型にCSTパラメータをフィッティングさせたりするpythonプログラムを作った
はじめに
CST(Class-Shape Transformation)法を使用して翼型の形状を生成したり、既存の翼型にCSTパラメータをフィッティングさせたりするpythonプログラムを作った
↓論文
↓参考
それではいってみよう
ソースコードの解説
import numpy as np
import pandas as pd
import matplotlib.pyplot as plt
from scipy.optimize import minimize
import random
import math
from scipy.interpolate import interp1d
class CST:
def __init__(self):
self.wl = None
self.wu = None
self.dz = None
self.Node = None
self.x = None
self.y = None
self.coord = None
self.x_old = None
self.y_old = None
self.xt = None
self.yt = None
self.ler = None
self.n_wl = None
self.n_wu = None
def _class_shape(self, w, x, N1, N2, dz):
C = x**N1 * (1 - x)**N2 # Class function
# Shape function; using Bernstein Polynomials
S = np.zeros_like(x)
nw = w.shape[1] - 1 # Order of Bernstein polynomials
K = np.zeros(nw+1)
for i in range(0, nw+1):
K[i] = math.factorial(nw)/(math.factorial(i)*(math.factorial((nw)-(i))))
for j in range(0, nw+1):
S += (w[:,j]*K[j]).reshape(-1,1)*x**(j) * ((1-x)**(nw-(j)))
y = C * S + x * dz
return y
def create_airfoil(self, wl=[[-1, -1, -1]], wu=[[1, 1, 1]], dz=[0], Node=100):
self.wl = np.array(wl)
self.wu = np.array(wu)
self.dz = np.array(dz).reshape(-1,1)
self.Node = Node
N = self.dz.shape[0]
if self.x is None: # Create x coordinate
self.x = np.ones((N,self.Node))
zeta = np.zeros((N,self.Node))
for i in range(self.Node):
zeta[:, i] = 2 * np.pi / (self.Node - 1)*i
self.x[:, i] = 0.5 * (np.cos(zeta[:, i])+ 1)
# Ni and N2 parameters (N1 = 0.5 and N2 = 1 for airfoil shape)
N1 = 0.5
N2 = 1
center_loc = np.argmin(self.x)
xu = self.x[:,:center_loc]
xl = self.x[:,center_loc:]
yu = self._class_shape(self.wu, xu, N1, N2, self.dz)
yl = self._class_shape(self.wl, xl, N1, N2, -self.dz)
self.x = np.concatenate([xu, xl],axis=1).reshape(N,-1)
self.y = np.concatenate([yu, yl],axis=1).reshape(N,-1)
self.coord = np.stack([self.x, self.y],axis=1)
return self.coord
def write_to_file(self, file_name = 'airfoil_shape.csv', N=0):
df = pd.DataFrame(self.coord[N].T, columns=['x', 'y'])
df.to_csv(file_name, header=True, index=False)
def fit_CST(self, file_name = 'airfoil_shape.csv', n_wl=4, n_wu=4):
data = pd.read_csv(file_name)
self.x_old = data['x'].to_numpy()
self.y_old = data['y'].to_numpy()
self.Node = len(data)
self.dz = [abs(self.y_old[-1])]
self.n_wl = n_wl
self.n_wu = n_wu
# normalization
self.x_old = self.x_old/(np.max(self.x_old)-np.min(self.x_old))
self.x_old = self.x_old-np.min(self.x_old)
self.y_old = self.y_old/(np.max(self.x_old)-np.min(self.x_old))
self.x = self.x_old.reshape(1,-1)
initial_guess = [random.uniform(-1,1) for _ in range(self.n_wl+self.n_wu)]
result = minimize(self._objfunc, initial_guess) # minimize
self.x=None
self.create_airfoil(wl=self.wl, wu=self.wu, dz=self.dz, Node=self.Node)
def _objfunc(self, x):
wl = [x[:self.n_wl]]
wu = [x[self.n_wl:self.n_wl + self.n_wu]]
self.create_airfoil(wl=wl, wu=wu, dz=[0], Node=self.Node)
f = np.sum((self.y * 100 - self.y_old * 100)**2)
return f
def get_var(self):
return self.wu, self.wl
def get_thickness(self):
thickness = []
x_tmax = []
self.xt = np.zeros_like(self.x)
self.yt = np.zeros_like(self.y)
for N in range(self.coord.shape[0]):
center_loc = np.argmin(self.coord[N,0])
coord_u = self.coord[N, :, :center_loc+1]
coord_l = self.coord[N, :, center_loc:]
fl = interp1d(coord_l[0,:], coord_l[1,:], kind= 'linear')
self.xt[N,:center_loc+1] = coord_u[0,:]
self.yt[N,:center_loc+1] = coord_u[1,:]-fl(coord_u[0,:])
fu = interp1d(coord_u[0,:], coord_u[1,:], kind='linear')
self.xt[N,center_loc:] = coord_l[0,:]
self.yt[N,center_loc:] = fu(coord_l[0,:])-coord_l[1,:]
thickness.append(np.max(self.yt[N]))
x_tmax.append(self.xt[N,np.argmax(self.yt[N])])
return np.array(thickness),np.array(x_tmax)
def get_ler(self):
if self.yt is None:
self.get_thickness()
self.ler = np.zeros(self.coord.shape[0])
for N in range(self.coord.shape[0]):
x0 = np.min(self.xt[N])
y0 = self.yt[N,np.argmin(self.xt[N])]/2
r = ((self.xt[N]-x0)**2+(-y0)**2)**0.5
center_loc = np.argmin(self.xt[N])
for i in range(center_loc,r.shape[0]):
d = ((self.xt[N,:]-self.xt[N,i])**2+(self.yt[N,:]/2-y0)**2)**0.5
if r[i]>np.min(d):
self.ler[N] = r[i-1]
break
return self.ler
def get_TE_angle(self):
if self.yt is None:
self.get_thickness()
TE_angle = []
for N in range(self.coord.shape[0]):
center_loc = np.argmin(self.coord[N,0])
f = interp1d(self.xt[N,:center_loc+1], self.yt[N,:center_loc+1], kind= 'linear')
x90 = 0.90
x99 = 0.99
y90 = f(x90)
y99 = f(x99)
TE_angle.append(math.degrees(math.atan2(abs(y90-y99),abs(x90-x99))))
return np.array(TE_angle)
def get_section_area(self):
if self.yt is None:
self.get_thickness()
x_diff = np.abs(np.diff(self.xt,n=1,axis=1))
section_area = np.sum(x_diff * self.yt[:,1:],axis=1)/2
return section_area
def plot(self,N=0):
fig, ax = plt.subplots()
ax.plot(self.x[N], self.y[N],label='CST')
if self.y_old is not None:
ax.plot(self.x_old, self.y_old,label='original')
if self.ler is not None:
theta = np.linspace(0, 2 * np.pi, 100)
#中心と半径から円の座標を計算
x = self.ler[N] + self.ler[N] * np.cos(theta)
y = self.ler[N] * np.sin(theta)
ax.plot(x, y,label='ler')
ax.plot(self.xt[N], self.yt[N]/2,label='Symmetry')
ax.set_aspect("equal", "box")
ax.set_xlim(0, 1)
ax.set_ylim(-0.5, 0.5)
ax.set_xlabel( 'x/c')
ax.set_ylabel( 'y/c')
ax.spines[ 'right'] .set_visible(False)
ax.spines[ 'top'] .set_visible(False)
ax.yaxis.set_ticks_position( 'left')
ax.xaxis.set_ticks_position( 'bottom')
ax.legend()
ax.plot
plt.show()
if __name__ == '__main__':
airfoil = CST()
airfoil.fit_CST(file_name='./CST/NACA4412.csv')
thickness, x_tmax = airfoil.get_thickness()
ler = airfoil.get_ler()
TE_angle = airfoil.get_TE_angle()
section_area = airfoil.get_section_area()
airfoil.plot(N=0)
wu = airfoil.wu
wl = airfoil.wl
airfoil.write_to_file()
print(wu,wl)
print(thickness,x_tmax,ler,TE_angle)
print(section_area)
このプログラムは、CST(Class-Shape Transformation)法を使用して翼型の形状を生成したり、既存の翼型に対してCSTパラメータをフィッティングさせたりするクラスを実装している
また、翼型の幾何学的特性を計算し、プロットするための機能も含まれている
このプログラムはのちのち最適化に使うことを想定しているため、バッチ処理に対応しており、すべての変数は[:, n]
のような二次元以上の配列になっている
依存ライブラリ
プログラムは以下のライブラリに依存している
- numpy
- pandas
- matplotlib
- scipy
- random
- math
CST
クラス
__init__
メソッド
def __init__(self):
self.wl = None
self.wu = None
self.dz = None
self.Node = None
self.x = None
self.y = None
self.coord = None
self.x_old = None
self.y_old = None
self.xt = None
self.yt = None
self.ler = None
self.n_wl = None
self.n_wu = None
引数: なし
処理:
CSTクラスのインスタンスを初期化し、翼型のパラメータと座標を格納するための属性を定義する
wl
,wu
: 下側と上側のCSTパラメータdz
: 翼型の後縁厚さNode
: 翼型の節点数x
,y
: 翼型のx座標とy座標coord
: 翼型の座標x_old
,y_old
: ベース翼型のx座標とy座標xt
,yt
: 厚みの計算に使用するx座標とy座標ler
: 前縁半径n_wl
,n_wu
: 下側と上側のCSTパラメータの数
返り値: なし
_class_shape
メソッド
def _class_shape(self, w, x, N1, N2, dz):
C = x**N1 * (1 - x)**N2 # Class function
# Shape function; using Bernstein Polynomials
S = np.zeros_like(x)
nw = w.shape[1] - 1 # Order of Bernstein polynomials
K = np.zeros(nw+1)
for i in range(0, nw+1):
K[i] = math.factorial(nw)/(math.factorial(i)*(math.factorial((nw)-(i))))
for j in range(0, nw+1):
S += (w[:,j]*K[j]).reshape(-1,1)*x**(j) * ((1-x)**(nw-(j)))
y = C * S + x * dz
return y
引数:
w
: ndarray - CSTパラメータx
: ndarray - x座標N1
: float - クラス関数のパラメータ1N2
: float - クラス関数のパラメータ2dz
: float - 翼型の後縁厚さ
処理:
- クラス関数
C
をx**N1 * (1 - x)**N2
で計算 - 形状関数
S
を計算 C
とS
を組み合わせてy
を計算
返り値:
y
: ndarray - 計算されたy座標
create_airfoil
メソッド
def create_airfoil(self, wu=[[-1, -1, -1]], wl=[[1, 1, 1]], dz=[0], Node=101):
self.wl = np.array(wl)
self.wu = np.array(wu)
self.dz = np.array(dz).reshape(-1,1)
self.Node = Node
N = self.dz.shape[0]
if self.x is None: # Create x coordinate
self.x = np.ones((N,self.Node))
zeta = np.zeros((N,self.Node))
for i in range(self.Node):
zeta[:, i] = 2 * np.pi / (self.Node - 1)*i
self.x[:, i] = 0.5 * (np.cos(zeta[:, i])+ 1)
# Ni and N2 parameters (N1 = 0.5 and N2 = 1 for airfoil shape)
N1 = 0.5
N2 = 1
center_loc = np.argmin(self.x)
xu = self.x[:,:center_loc]
xl = self.x[:,center_loc:]
yu = self._class_shape(self.wu, xu, N1, N2, self.dz)
yl = self._class_shape(self.wl, xl, N1, N2, -self.dz)
self.x = np.concatenate([xu, xl],axis=1).reshape(N,-1)
self.y = np.concatenate([yu, yl],axis=1).reshape(N,-1)
self.coord = np.stack([self.x, self.y],axis=1)
return self.coord
引数:
wl
: list - 下面のCSTパラメータwu
: list - 上面のCSTパラメータdz
: list - 翼型の後縁厚さNode
: int - x座標の分割数
処理:
- CSTパラメータと後縁厚さを設定
- x座標を計算
- クラス関数と形状関数を用いて上面と下面のy座標を計算
- 上面と下面の座標を結合して翼型の座標を生成
返り値:
self.coord
: ndarray - 生成された翼型の座標
節点数が奇数なら(0,0)に前縁点を持ってくることができる
write_to_file
メソッド
def write_to_file(self, file_name = 'airfoil_shape.csv', N=0):
df = pd.DataFrame(self.coord[N].T, columns=['x', 'y'])
df.to_csv(file_name, header=True, index=False)
引数:
file_name
: str - 保存するファイル名N
: int - 保存する翼型のインデックス
処理:
self.coord
から指定された翼型の座標を取得- DataFrameに変換し、CSVファイルに保存
返り値: なし
fit_CST
メソッド
def fit_CST(self, file_name = 'airfoil_shape.csv', n_wl=4, n_wu=4):
data = pd.read_csv(file_name)
self.x_old = data['x'].to_numpy()
self.y_old = data['y'].to_numpy()
self.Node = len(data)
self.dz = [abs(self.y_old[-1])]
self.n_wl = n_wl
self.n_wu = n_wu
# normalization
self.x_old = self.x_old/(np.max(self.x_old)-np.min(self.x_old))
self.x_old = self.x_old-np.min(self.x_old)
self.y_old = self.y_old/(np.max(self.x_old)-np.min(self.x_old))
self.x = self.x_old.reshape(1,-1)
initial_guess = [random.uniform(-1,1) for _ in range(self.n_wl+self.n_wu)]
result = minimize(self._objfunc, initial_guess) # minimize
self.x=None
self.create_airfoil(wl=self.wl, wu=self.wu, dz=self.dz, Node=self.Node)
引数:
file_name
: str - 翼型データのCSVファイル名n_wl
: int - 下面のCSTパラメータの数n_wu
: int - 上面のCSTパラメータの数
処理:
- CSVファイルから対象の翼型データを読み込む
- x座標が前縁(0,0)、後縁(1,0)になるようにデータを正規化する
- 最適化の初期値を設定する(-1から1の範囲で適当に生成した乱数)
scipy.optimize
の最適化メソッドminimize
を使用して、元の翼型と生成した翼型の二乗和誤差が最小になるようにCSTパラメータを求める- 最適化されたCSTパラメータを使用して翼型を再生成する
返り値: なし
↓参考
_objfunc
メソッド
def _objfunc(self, x):
wl = [x[:self.n_wl]]
wu = [x[self.n_wl:self.n_wl + self.n_wu]]
self.create_airfoil(wl=wl, wu=wu, dz=[0], Node=self.Node)
f = np.sum((self.y * 100 - self.y_old * 100)**2)
return f
このプライベートメソッドは、fit_CST
メソッドにおいて元の翼型と生成した翼型の二乗和誤差が最小になるようにCSTパラメータを求める際に用いられる
引数:
x
: list - 最適化のための変数
処理:
- 下側と上側のCSTパラメータを設定する
- 与えられたCSTパラメータで翼型を生成する
- 生成された翼型と元の翼型との誤差を計算する
返り値:
f
: float - 誤差の二乗和
get_var
メソッド
def get_var(self):
return self.wu, self.wl
引数: なし
処理: 上面と下面の現在のCSTパラメータを取得
返り値:
self.wu
: ndarray - 上面のCSTパラメータself.wl
: ndarray - 下面のCSTパラメータ
get_thickness
メソッド
def get_thickness(self):
thickness = []
x_tmax = []
self.xt = np.zeros_like(self.x)
self.yt = np.zeros_like(self.y)
for N in range(self.coord.shape[0]):
center_loc = np.argmin(self.coord[N,0])
coord_u = self.coord[N, :, :center_loc+1]
coord_l = self.coord[N, :, center_loc:]
fl = interp1d(coord_l[0,:], coord_l[1,:], kind= 'linear')
self.xt[N,:center_loc+1] = coord_u[0,:]
self.yt[N,:center_loc+1] = coord_u[1,:]-fl(coord_u[0,:])
fu = interp1d(coord_u[0,:], coord_u[1,:], kind='linear')
self.xt[N,center_loc:] = coord_l[0,:]
self.yt[N,center_loc:] = fu(coord_l[0,:])-coord_l[1,:]
thickness.append(np.max(self.yt[N]))
x_tmax.append(self.xt[N,np.argmax(self.yt[N])])
return np.array(thickness),np.array(x_tmax)
引数: なし
処理:
- 翼型について、上面と下面の厚みを計算
- 最大厚みとその位置を計算
返り値:
thickness
: ndarray - 翼厚の配列x_tmax
: ndarray - 最大翼厚の位置の配列
get_ler
メソッド
def get_ler(self):
if self.yt is None:
self.get_thickness()
self.ler = np.zeros(self.coord.shape[0])
for N in range(self.coord.shape[0]):
x0 = np.min(self.xt[N])
y0 = self.yt[N,np.argmin(self.xt[N])]/2
r = ((self.xt[N]-x0)**2+(-y0)**2)**0.5
center_loc = np.argmin(self.xt[N])
for i in range(center_loc,r.shape[0]):
d = ((self.xt[N,:]-self.xt[N,i])**2+(self.yt[N,:]/2-y0)**2)**0.5
if r[i]>np.min(d):
self.ler[N] = r[i-1]
break
return self.ler
引数: なし
処理:
self.yt
が未計算の場合、get_thickness
を呼び出して翼厚を計算- 各翼型について、前縁半径を計算
返り値:
self.ler
: ndarray - 前縁半径の配列
節点数が少ないと露骨に精度が落ちるので、節点数は200点くらいあると安心
get_TE_angle
メソッド
def get_TE_angle(self):
if self.yt is None:
self.get_thickness()
TE_angle = []
for N in range(self.coord.shape[0]):
center_loc = np.argmin(self.coord[N,0])
f = interp1d(self.xt[N,:center_loc+1], self.yt[N,:center_loc+1], kind= 'linear')
x96 = 0.96
x99 = 0.99
y96 = f(x96)
y99 = f(x99)
TE_angle.append(math.degrees(math.atan2(abs(y96-y99),abs(x96-x99))))
return np.array(TE_angle)
引数: なし
処理:
self.yt
が未計算の場合、get_thickness
を呼び出して翼厚を計算- 翼型について、後縁角を計算
返り値:
TE_angle
: ndarray - 後縁角の配列
get_section_area
メソッド
def get_section_area(self):
if self.yt is None:
self.get_thickness()
x_diff = np.abs(np.diff(self.xt,n=1,axis=1))
section_area = np.sum(x_diff * self.yt[:,1:],axis=1)/2
return section_area
引数: なし
処理:
self.yt
が未計算の場合、get_thickness
を呼び出して翼厚を計算- 翼型について、断面積を計算
返り値:
section_area
: ndarray - 断面積の配列
plot
メソッド
def plot(self,N=0):
fig, ax = plt.subplots()
ax.plot(self.x[N], self.y[N],label='CST')
if self.y_old is not None:
ax.plot(self.x_old, self.y_old,label='original')
if self.ler is not None:
theta = np.linspace(0, 2 * np.pi, 100)
#中心と半径から円の座標を計算
x = self.ler[N] + self.ler[N] * np.cos(theta)
y = self.ler[N] * np.sin(theta)
ax.plot(x, y,label='ler')
ax.plot(self.xt[N], self.yt[N]/2,label='Symmetry')
ax.set_aspect("equal", "box")
ax.set_xlim(0, 1)
ax.set_ylim(-0.5, 0.5)
ax.set_xlabel( 'x/c')
ax.set_ylabel( 'y/c')
ax.spines[ 'right'] .set_visible(False)
ax.spines[ 'top'] .set_visible(False)
ax.yaxis.set_ticks_position( 'left')
ax.xaxis.set_ticks_position( 'bottom')
ax.legend()
ax.plot
plt.show()
引数:
N
: int - プロットする翼型のインデックス
処理:
- 指定された翼型をプロット
- フィッティングした際に、元の翼型データが存在する場合、それもプロット
- 前縁半径と仮想的な対称翼型をプロット
返り値: なし
メインプログラム
if __name__ == '__main__':
airfoil = CST()
airfoil.fit_CST(file_name='./CST/NACA4412.csv')
thickness, x_tmax = airfoil.get_thickness()
ler = airfoil.get_ler()
TE_angle = airfoil.get_TE_angle()
section_area = airfoil.get_section_area()
airfoil.plot(N=0)
wu = airfoil.wu
wl = airfoil.wl
print(wu,wl)
print(thickness,x_tmax,ler,TE_angle)
print(section_area)
airfoil.write_to_file()
このセクションは、スクリプトとして実行されたときに実行される
CST
クラスのインスタンスを作成するfit_CST
メソッドを使用して、指定されたCSVファイルから翼型データを読み込み、CSTパラメータにフィッティングさせる- 各種メソッドを使用して翼型の形状を取得する
plot
メソッドを使用して翼型をプロットする- 結果をCSVファイルに保存し、計算結果を表示する
使い方
CSTパラメータをフィッティングさせたい翼型を.csvファイルにして、CST.pyと同じディレクトリに置く
__main__
関数の中のairfoil.fit_CST()
の引数のfile_name
を適当に書き換える
airfoil.fit_CST(file_name='./NACA4412.csv')
あとはターミナルで以下のコマンドを実行する
> py -3.10 CST.py
標準出力としてCSTパラメータなどが表示されるので、あとは煮るなり焼くなり好きにすればいい
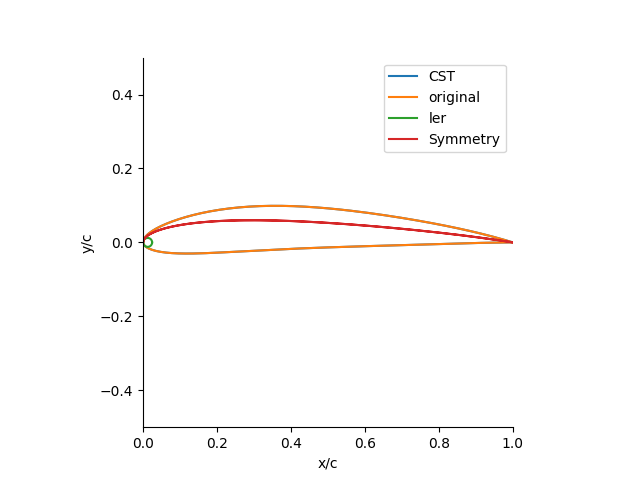
↓最適化で使いたい場合はこっちを参照
おわりに
CST(Class-Shape Transformation)法を使用して翼型の形状を生成したり、既存の翼型にCSTパラメータをフィッティングさせたりするpythonプログラムを作った
翼型の形状を少ないパラメータ数でいい感じに表現できるので、最適化なりモデリングなりで楽しく使えそうである
↓関連記事
コメント